Bayesians Intriguing from Defense to Offense
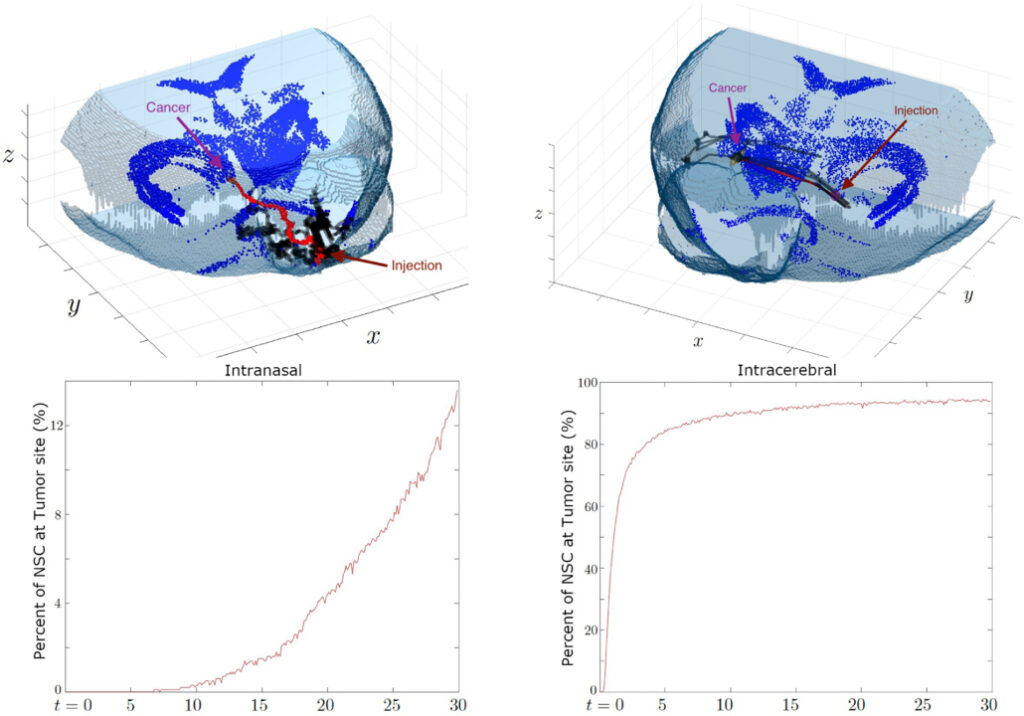
Erik van Zwet, Sander Greenland, Guido Imbens, Simon Schwab, Steve Goodman, and I write:
Now we enjoy examined the first efficacy outcomes of 23,551 randomized scientific trials from the Cochrane Database of Systematic Reports.
We estimate that the substantial majority of trials enjoy distinguished decrease statistical energy for staunch effects than the 80 or 90% for the said finish sizes. As a consequence, “statistically main” estimates are inclined to severely overestimate staunch drugs effects, “nonsignificant” outcomes typically correspond to extensive effects, and efforts to copy typically fail to finish “significance” and can also even appear to contradict preliminary outcomes. To tackle these components, we reinterpret the P rate in phrases of a reference inhabitants of learn that are, or may perchance were, within the Cochrane Database.
This ends in an empirical recordsdata for the interpretation of an observed P rate from a “conventional” scientific trial in phrases of the extent of overestimation of the reported finish, the likelihood of the finish’s signal being immoral, and the predictive energy of the trial.
Such an interpretation presents extra insight about the finish below witness and may perchance guard scientific researchers against naive interpretations of the P rate and overoptimistic finish sizes. Because many learn fields endure from low energy, our outcomes are also related originate air the scientific domain.
Also this new paper from Zwet with Lu Tian and Rob Tibshirani:
Evaluating a shrinkage estimator for the drugs finish in scientific trials
The first goal of most scientific trials is to estimate the finish of some drugs in contrast to a retain watch over condition. We give an explanation for the signal-to-noise ratio (SNR) as the ratio of the precise drugs finish to the SE of its estimate. In a old newsletter on this journal, we estimated the distribution of the SNR among the many scientific trials within the Cochrane Database of Systematic Reports (CDSR). We found out that the SNR is basically low, which capacity that that the energy against the precise finish will be low in many trials. Right here we exercise the truth that the CDSR is a series of meta-analyses to quantitatively assess the penalties. Amongst trials which enjoy reached statistical significance we uncover mighty overoptimism of the same old self ample estimator and below-coverage of the related confidence interval. Previously, now we enjoy got proposed a unusual shrinkage estimator to address this “winner’s curse.” We evaluate the efficiency of our shrinkage estimator to the same old self ample estimator in phrases of the inspiration mean squared error, the coverage and the bias of the magnitude. We uncover superior efficiency of the shrinkage estimator each conditionally and unconditionally on statistical significance.
Let me dazzling repeat that final sentence:
We uncover superior efficiency of the shrinkage estimator each conditionally and unconditionally on statistical significance.
From a Bayesian standpoint, this is never any surprise. Bayes is optimum must you average over the prior distribution and can also furthermore be practical if averaging over something shut to the prior. Particularly practical in contrast to naive unregularized estimates (as here).
Erik summarizes:
We’ve sure how distinguished we create (on average over the Cochrane Database) by utilizing our shrinkage estimator. It appears to be like to be just a few component 2 more atmosphere pleasant (in phrases of the MSE) than the self ample estimator. That’s roughly care for doubling the pattern dimension! We’re using equivalent suggestions as our forthcoming paper about meta-prognosis with a single trial.
Of us in most cases query me how I’ve changed as a statistician over time. One answer I’ve given is that I’ve step by step become more Bayesian. I started out as a skeptic, aroused by Bayesian suggestions at all; then in grad college I started using Bayesian statistics in capabilities and realizing it may perchance perchance perchance solve some issues for me; when writing BDA and ARM, mute having the Bayesian balk and using flat priors as distinguished as possible, or now not speaking about priors at all; then with Alex, Sophiaand others transferring toward weakly informative priors; at final below the impact of Erik and others in search of to exercise assert prior knowledge. At this level I’ve magnificent distinguished long past corpulent Lindley.
Right as a comparison to the salvage my colleagues and I are now, inspect my response in 2008 to a request from Sanjay Kaul about suggestions on how to specify a old distribution for a scientific trial. I wrote:
I affirm the most attention-grabbing prior distribution may perchance well be in step with a multilevel model (whether implicit or explicit) in step with completely different, equivalent experiments. A noninformative prior can also very effectively be okay however I prefer something weakly informative to retain away from your inferences being unduly plagued by extraordinarily unrealistic probabilities within the tail of the distribuiton.
Nothing immoral with this advice, exactly, however I become as soon as mute leaning within the route of noninformativeness in a capacity that I’d now not anymore. Sander Greenland replied on the time with a advice to exercise assert prior knowledge. (And, dazzling for fun, here’s a dialogue from 2014 on a subject subject the salvage Sander and I disagree.)
Erik concludes:
I truly affirm it’s form of irresponsible now now not to exercise the realizing from all those thousands of scientific trials that came sooner than. Is that very radical?
That final request strikes a chord in my memory of our paper from 2008, Bayes: Radical, Liberal, or Conservative?
P.S. Also this:
You possible can click thru to ogle the total story.